Text Summarization with AI: Exploring Uses and Methods
Last Updated on
May 31, 2024
Published:
May 6, 2024
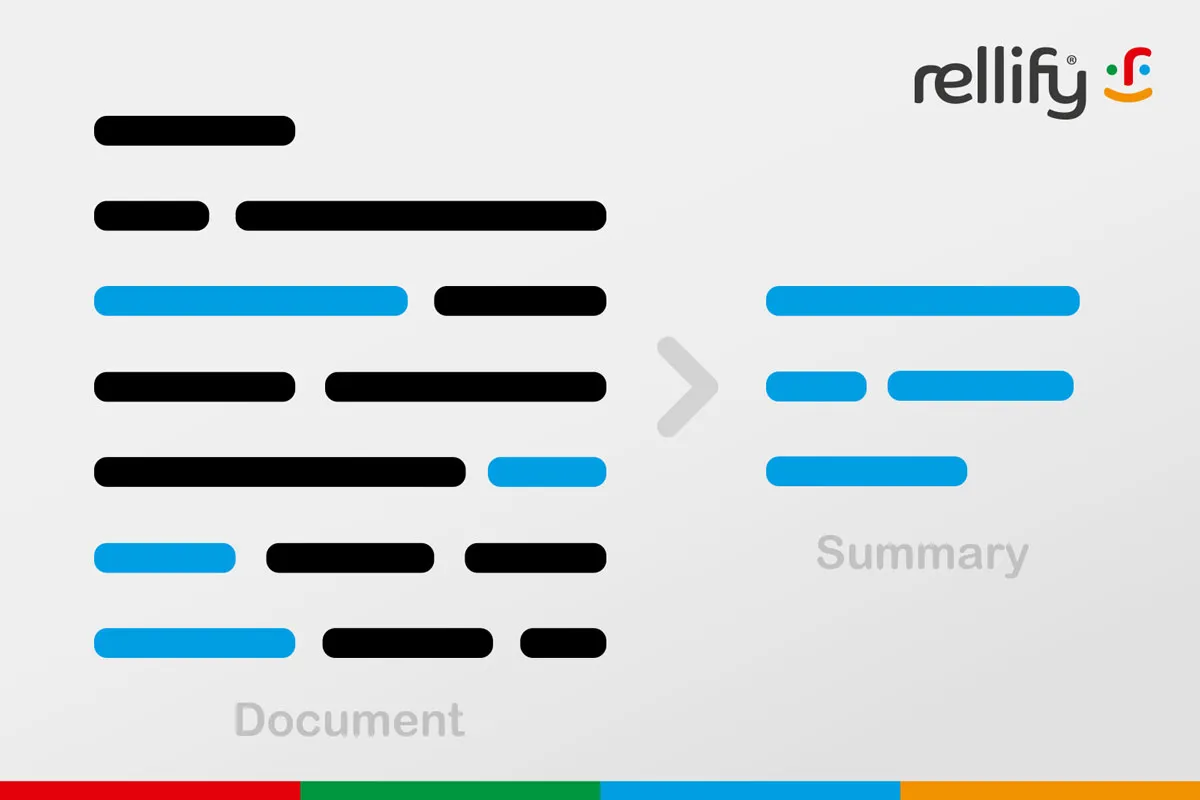
By Jayne Schultheis - The process of distilling the key points or main ideas from a piece of text while preserving its essence is called text summarization. Children learn how to do it in elementary school; it's a key part of reading comprehension. Through artificial intelligence (AI), computers can manage the task as well.
Text summarization algorithms condense longer documents or segments of text into shorter versions, usually by identifying important sentences or passages and presenting them coherently and concisely. The summaries help users to quickly understand the content of a document.
Text summarization with AI is having a major impact in many business sectors, including content marketing. In this article, we'll focus on how this new capability is helping marketers streamline the content creation process.
How does AI use text summarization technology?
The main branches of AI used for summarization techniques and approaches are natural language processing (NLP), natural language generation (NLG), and natural language understanding (NLU). Let's look at some different forms of automatic summarization.
Extractive summarization
NLP tools like GPT and Google's BERT use this method of text processing. After data preprocessing, extractive summarization methods select and extract important sentences or phrases directly from the input text without generating new sentences.
TF-IDF (Term Frequency-Inverse Document Frequency) is commonly used as part of extractive summarization. TF-IDF is a measure of the importance of a word in a document relative to a collection of documents or pages. It is commonly used in information retrieval and text mining to identify the relevance of a word to a document.
Real-world example: In a news article about a recent event, an extractive summarization algorithm might identify the sentences containing the most frequently occurring keywords or phrases and select them for a summary.
Abstractive summarization
Abstractive summarization methods generate a summary by interpreting and rephrasing the content of the input text in a more concise form.
This approach involves understanding the meaning of the text and generating novel sentences to convey the summarized information.
Real-world example: An abstractive summarization model might read a product review and generate a summary that captures the sentiments and key aspects of the review in new, condensed sentences.
Query-based summarization
Query-based summarization, or summary generation, is based on specific user queries or questions, combining elements of NLP and NLU.
NLU involves analyzing and interpreting text to comprehend its meaning and extract relevant information. This is achieved through techniques like tokenization, parsing, named entity recognition, semantic analysis, and sentiment analysis.An NLU model learns to understand the context and relationships between words and phrases in text, as well as the overall meaning of the content. Query-based summarization can be done using extractive or abstractive summarization models.
Real-world example: A query-based summarization system might analyze a medical research paper and generate a summary focused on answering a specific medical question posed by a user.
Multi-document summarization
Sometimes, you might have a lot of info in a lot of different places that all need to be scanned and summarized.
Multi-document summarization assesses multiple documents on a given topic. AI systems use techniques such as clustering, topic modeling, and information fusion to identify common themes and extract key information from multiple sources.
Real-world example: A news aggregation platform that collects sports articles gives users with a concise summary of each one so they can get a quick overview of the sports world.
How does machine learning improve text summarization?
Machine learning significantly improves text summarization in many ways. Here are three important ones:
- Sequence-to-sequence models. Sequence-to-sequence models, particularly transformer-based architectures like BERT and GPT, have revolutionized text summarization by allowing models to generate summaries of different lengths directly from input text. These models can capture complex relationships and dependencies in text, which means high-quality summaries.
- Attention mechanisms. Attention mechanisms allow models to focus on relevant parts of the input text when generating summaries. Models equipped with attention mechanisms can better capture important information and produce coherent and relevant summaries with contextual understanding.
- Data-driven approaches. Machine learning models are trained on diverse, big datasets. Data-driven approaches allow models to learn patterns and relationships in the data, leading to improved performance in text summarization tasks. By training on relevant datasets, models can better understand the nuances of language and generate more accurate summaries.
How text summarization with AI can boost your content marketing efforts
Content marketing strives to be relevant and to make its points quickly. Automatic summarization makes it easier to achieve both goals. Here are some ways that content marketers are using text summarization.
Streamlined content consumption
By providing concise summaries of your content, AI-powered text summarization helps readers to quickly grasp the main points and key takeaways without having to read the entire article or document. This improves content consumption rates and enhances the overall user experience.
Increased engagement
Summarized content is more likely to capture the attention of busy readerswho may not have the time to read lengthy articles. By delivering the most important information upfront, AI-generated summaries can increase engagement and encourage users to go deeper into your content and stay on your site longer.
Enhanced SEO
Search engines value content that is relevant, informative, and easy to digest. By incorporating AI-generated summaries into your content marketing strategy, you can improve the readability and relevance of your content, leading to better search engine rankings and increased organic traffic. We'll talk more later about how text summarization connects to the process of topic ideation and keyword extraction within Rellify, thereby enhancing your SEO.
Efficient content curation
Content marketers often curate content from various sources to create comprehensive resources for their audience. AI-driven summarization tools can automate the process of summarizing and synthesizing content from multiple sources, saving time and effort in content curation.
Scalability
As your content marketing efforts grow, AI-powered text summarization can help you scale your content production and distribution efforts. Using automated summarization, you can generate summaries for a large volume of content quickly and efficiently. You'll be able to reach a broader audience.
How we do text summarization with AI at Rellify
Most of the text summarization methods we mentioned above are employed both within Rellify's content platform and in the Relliverse™ we can create for a client.
A Relliverse™ is a custom AI subject-matter expert that identifies your best content opportunities. We employ deep machine learning to crawl the websites of top competitors in your industry or niche, as well as your own and related sites. We analyze that data to find patterns in keywords or topics that are already ranking high on search engines, or that are not being addressed by your competition. This kind of topic modeling is an example of extractive summarization.
Rellify then uses semantic analysis to build meaningful connections and categorization between and within these topics, much like neural networks. The most meaningful and impactful topics and keywords are aggregated and presented to you, ready to be strategically used in content in ways that will boost your SEO.
In the Rellify platform, you have lots of opportunities to use text summarization with AI. When you build a brief, Rellify's AI capabilities use abstractive text summarization to categorize keywords and place them most effectively into an outline.
When you start creating content, Rellify's generative AI capabilities are ready to help. You can use it to generate an entire blog article, a high-quality summary of an article, or a meta description. You are in the driver's seat of content management as the editor. AI is merely at your disposal every step of the way.
Wondering about the Rellify platform and how a custom Relliverse can boost your content marketing ROI with significantly less effort? Contact a Rellify expert and get a demo of the platform and a custom Relliverse today!